Dr. Trinh began by defining artificial intelligence as the use of machine-learning algorithms and software to mimic human cognition in the analysis, presentation, and comprehension of complex medical and health care data. While artificial has been discussed since the 1940s, driven at that time by the concept of the Turing Machine, there have been significant computational advances and innovations in the past two decades which have brought it from a novelty to potentially a disruptive contributor to health care delivery.
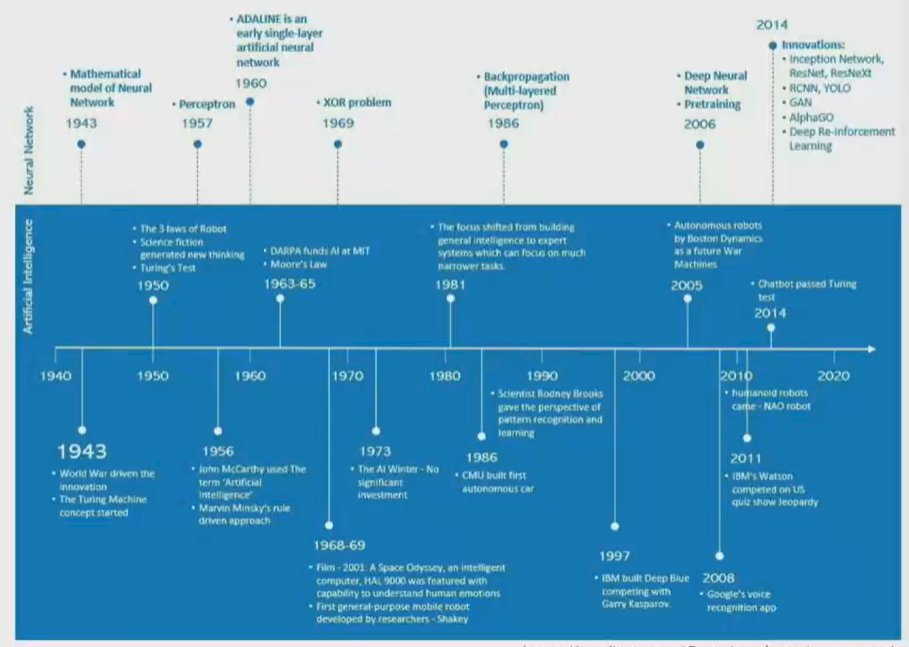
In this context, he highlighted the rationale for interest and focus on artificial intelligence now:
- The value challenge of health care delivery with escalating demands due to an aging population and rising costs
- An explosion in the amount of readily availably health data
- Advances in information technology and computational ability
- A democratization of access to data
- A willingness from the general public to consider these approaches.
Investment in artificial intelligence, particularly in North American and in the United States, have increased dramatically in the past few years and are anticipated to continue to rise at a nearly exponential rate.

The goal of AI in health care is to move from data to data-driven medical decisions. However, Dr. Trinh highlighted that there are many challenges in this paradigm. First, from an ethical perspective, we must consider the informed consent to use data, the importance of safety and transparency, algorithmic fairness and biases, and data privacy. From a legal perspective, we further need to consider safety and effectiveness, liability, data protection and privacy, cybersecurity, and intellectual property law. In this talk, he focused on fairness and bias as they relate to the safety and effectiveness of the models. He emphasized that AI can unintentionally lead to unjust outcomes through a variety of mechanisms including a lack of insight into sources of bias in the data and the model, a lack of insight into the feedback loops which may amplify biases, lack of careful disaggregated evaluation, and human biases in interpreting and accepting results.
In part, he emphasized that there is increasing complexity in both the data and interpretation of data the underpins health care delivery. Techniques, such as propensity scores, inverse probability of treatment weighting, instrumental variable analyses, and others, drawn from the health economics literature have added methodologic complexity to the medical literature. However, he suggested that these approaches remain within the contexts of what a clinician may understand. However, deep learning models are often substantially more complex than can be captured completely within a manuscript and, beyond this, maybe beyond what a clinician who is trying to apply information to a clinician scenario can understand.

Thus, for many clinicians, artificial intelligence represents a black box in which the derivation of a model predictive output is entirely opaque. While these predictions may be “better” than prior approaches, Dr. Trinh emphasized that understanding the working of these models is somewhat unclear.
Additionally, deep learning depends heavily on both the data fed into the model and the trainers who collect and annotate data and outcomes. Biases in either the data or the collection and annotation may lead to not just spurious, but potentially biased and harmful results.

Further, on the basis of built-in assumptions and reinforcement, machine learning may emphasize bias. Citing an example of images of cooking, he emphasized that while 67% of individuals who were cooking in the dataset were women, the AI-based algorithm predicted that 84% of individuals cooking were women. The feedback loop may serve to further potential algorithmic bias through a network effect. When model results are used to inform decisions which then serve to create data that is used to inform the model, biases in the initial training data and annotation may be perpetuated and magnified. This is a phenomenon known as algorithmic bias.
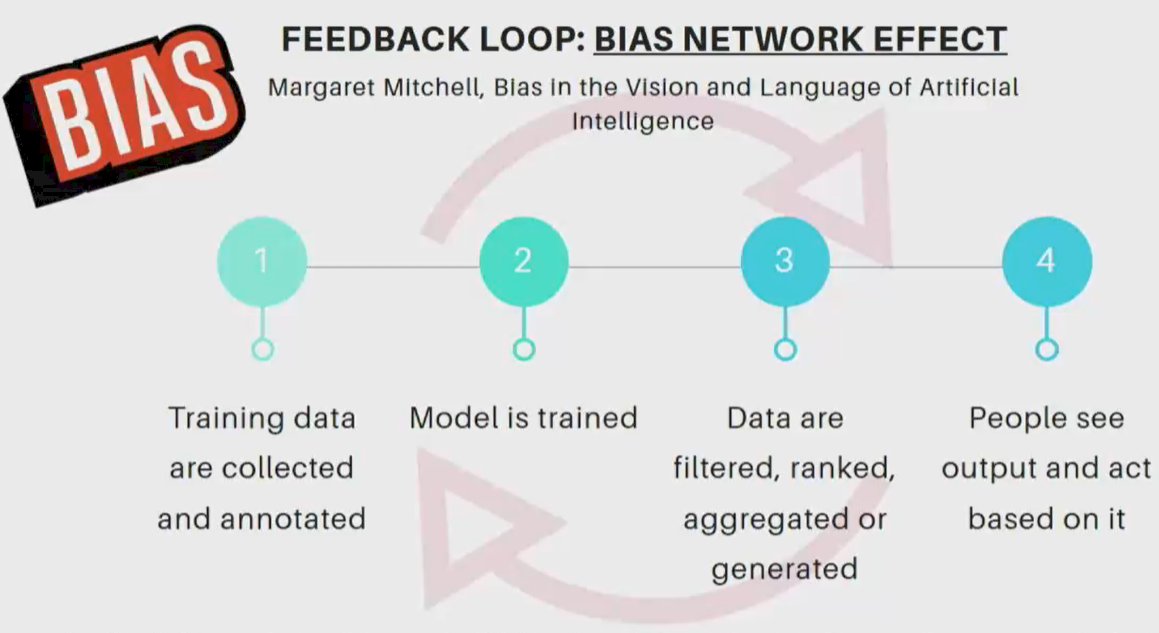
Dr. Trinh emphasized that, like many other advances before, medicine has been somewhat late in the uptake of AI. Citing an example from criminal justice, he emphasized the use of an algorithm that identifies individuals who are at a high likelihood of committing a crime. This information may lead to police departments proactively reaching out to individuals. These models were informed based on arrests for drug use which, as Dr. Trinh pointed out, may not reflect actual drug use and may have substantial underlying racial, or other, biases. Thus, police may be preferentially driven to focus on areas informed by biased data which may disproportionately affect Black individuals. Thus, arrests driven by a biased dataset or algorithm are likely to reinforce these biases and even further amplify them. These kind of algorithms may also be used to inform sentencing recommendations. Citing another example, he emphasized that biases in these AI-based approaches may serve to exacerbate disparities and biases which are existing in society. Thus, we need to be thoughtful, pragmatic, and careful of how we apply these.
Moving to a medical example, he highlighted that current eGFR calculations result in her estimation of eGFR for the same creatinine among Black individuals as compared to non-Blacks. This results in potentially higher drug dosing, delayed transplantation, later chronic kidney disease referrals, and later dialysis initiation. Each of these may serve to unduly restrict access to care. In this example, Dr. Trinh emphasized that the transparency of the model for calculating eGFR can allow for the identification of potential sources of biases. When AI-based models are somewhat of a “black box” it may be substantially more difficult to identify these issues.
Algorithms have been used to determine whether patients should receive home health services. As Black patients have historically incurred lower health care costs (for a variety of reasons) for the same medical conditions, the algorithm assigned a higher score to a White patient for the same medical severity as costs were used to assess the need for these services. As a results, White patients were more likely to be selected to receive additional home health care. This serves to further exacerbate disparities in access to care. However, the algorithm may be modified in a manner that reduces the disparity by more than 80% by predicting future costs and the number of times a chronic condition might flare up over the coming year, rather than relying only on historical inputs. He emphasized that this example highlights how the use of AI may create or exaggerate problems, rather than ameliorating them if we don’t understand the model and the predictions being made.
In the context of prostate cancer, he cited an example of assessing the prediction of suitability of active surveillance for prostate cancer. Currently, data shows that Black men have a higher prostate cancer mortality when treated with surveillance for Gleason 6 prostate cancer. However, rather than disease biology, at least some portion of this difference may be due to differences in care. Thus, an algorithm informed only by data demonstrating an increased risk of mortality among Black men may inappropriately classify Black men as being unsuitable for active surveillance, an effect which may potentiate worse outcomes in these men.
Dr. Trinh then discussed “statistical metrics of fairness”, emphasizing that we need to consider the big picture. First, we need to ensure that there needs to be equality of opportunity. Thus, models derived in limited populations may not be useful or valid when applied more generally. Certainly, depending on context, we may prioritize false positive or false negative results. Understanding these will allow clinicians to best operationalize AI in health care.

Dr. Trinb emphasized the importance of step 5 with validation and implementation, while not critical in the academic process, is critical in contributing to the development of technologies which may transform care.
While this presentation focused heavily on the potential for AI to potentiate bias, Dr. Trinh further highlighted other issues in terms of privacy issues and others.
Presented by: Quoc-Dien Trinh, MD, Associate Professor of Surgery, Harvard Medical School, Boston, MA